Abstract
Text generation, a field at the intersection of natural language processing (NLP) and artificial intelligence (AI), has witnessed profound advancements in recent years, driven by algorithms that can learn from vast quantities of text data. This observational research article explores the development of text generation technologies, the methodologies employed, and the implications for various sectors, including education, content creation, and customer service. By examining existing literature and conducting an observational study of text generation systems, this paper aims to provide a comprehensive overview of the current state of text generation and its future prospects.
Introduction
The ability to automatically generate coherent, contextually relevant text has been a significant technical hurdle and a goal for researchers in the fields of linguistics, computer science, and AI. Recent breakthroughs, particularly involving deep learning and transformer-based architectures such as OpenAI's GPT-3, have transformed the landscape of text generation. This field not only captivates researchers and developers but also influences industries and the broader public discourse on AI capabilities.
The growing reliance on automated text generation raises questions about the implications of these technologies for human creativity, ethical considerations, and the overall impact on language and communication. In this article, we participate in a systematic observation of various text generation technologies, analyze their methodologies, applications, and social ramifications, and offer insights for future research directions.
Methodology
To evaluate the current trends and technologies in text generation, this research adopts a multi-faceted approach. We conduct a review of the literature on text generation, coupled with an observational study aimed at gathering empirical data on the user experience and effectiveness of different text generation systems.
- Literature Review: Academic papers, case studies, and industry reports were analyzed to understand the evolution of text generation, key algorithms, methodologies, and applications.
- Observational Study: We assessed popular text generation tools including OpenAI's GPT-3, Google's BERT, and other emerging AI models. We focused on user interactions, contextual understanding, and text coherence in various applications, such as content creation, chatbots, and automated reporting.
- User Surveys and Interviews: Feedback was collected from a diverse group of users across different sectors to gauge their experiences and perceptions of text generation technologies.
Findings
1. The Evolution of Text Generation
Text generation technologies have evolved from rule-based systems and traditional statistical methods to highly sophisticated neural networks. Early approaches, such as ELIZA, relied on simple pattern matching and keyword recognition. As computational power increased, so did the complexity of these models.
The introduction of neural networks marked a shift towards more human-like text generation. Long Short-Term Memory (LSTM) networks and Recurrent Neural Networks (RNNs) paved the way for improved contextual understanding, and the advent of the Transformer architecture revolutionized the field. Transformers allow parallel processing of data, enabling greater efficiency and scalability.
2. Current Architectures and Algorithms
The following key architectures are significant in the field of text generation:
- Generative Pre-trained Transformers (GPT): These models are pre-trained on diverse internet text before being fine-tuned ChatGPT for marketing - http://josefllnm300-konverzace.lowescouponn.com/jak-se-vyhnout-beznym-chybam-pri-praci-s-chatgpt-4, specific tasks, allowing them to generate human-like text based on minimal input.
- Bidirectional Encoder Representations from Transformers (BERT): While primarily used for understanding context and sentiment, BERT's ability to analyze and produce text has contributed to text generation capabilities in chatbots and other applications.
- Variational Autoencoders (VAEs) and Generative Adversarial Networks (GANs): These methods introduce a nuanced approach where one network generates text while another evaluates its quality, improving the coherence and creativity of the output.
3. Applications of Text Generation
The applications of text generation are vast and continue to expand rapidly:
- Content Creation: Organizations employ text generation systems for blog posts, product descriptions, and news articles. This automation not only saves time but can lead to content that is tailored to audience preferences.
- Customer Support: Chatbots utilize text generation algorithms to respond to inquiries quickly and accurately. The ability to provide contextual responses helps enhance customer satisfaction.
- Personalized Marketing: Companies are adopting text generation to create personalized emails and promotional content based on user behavior and preferences, increasing engagement and conversion rates.
- Language Translation: Advanced text generation models have improved machine translation, making it more natural and contextually relevant.
4. Challenges and Limitations
Despite its remarkable advancements, text generation technology faces several challenges:
- Coherence and Context: While modern models generate text that appears coherent at first glance, maintaining contextual accuracy over longer passages remains a significant challenge.
- Bias and Ethical Concerns: Generated text may inadvertently reflect biases present in the training data, raising ethical questions regarding discrimination and misinformation.
- Dependence on Data Quality: The effectiveness of text generation systems directly correlates to the quality and diversity of the training data. A lack of representation can lead to subpar performance in generating inclusive content.
5. User Experiences and Observations
Throughout our observational study, we interacted with several users and gathered insights on their experiences with text generation technologies:
- Ease of Use: Users generally found text generation tools intuitive and user-friendly, providing a low barrier for entry for non-technical users.
- Content Quality: While many users were impressed by the output quality, some expressed skepticism regarding the originality of generated content, noting a tendency for repetition and cliché phrasing.
- Enhancement of Creativity: Several content creators reported using text generators as collaborative tools to spark ideas and enhance creativity, rather than as mere replacements for human creativity.
- Ethical Considerations: Users voiced concerns about the ethical implications of using AI-generated text, particularly in areas like journalism and academic writing, where authenticity and originality are crucial.
Discussion
The observation of text generation technologies has illuminated both the significant potential and the challenges that lie ahead. As these technologies continue to develop, they will play an increasingly critical role in various sectors. However, with great power comes great responsibility.
Concerns regarding bias, misinformation, and ethical usage must be addressed through transparent training processes, diverse datasets, and ongoing research into the ethical implications of AI-generated content. Educational institutions and organizations should prioritize guidelines and transparency in deploying these technologies.
Future Research Directions
Future research in text generation should focus on several key areas:
- Bias Mitigation: Developing techniques for identifying and reducing bias in AI-generated text to ensure fairness and inclusivity.
- Improving Coherence: Continuing to refine algorithms to enhance the contextual understanding and coherence of generated output over long passages.
- Interactive AI: Exploring the integration of human feedback into the generation process to improve contextual responsiveness and relevance.
- Regulatory Frameworks: Advocating for policies that govern the ethical use of text generation technologies across different sectors.
- User Education: Promoting awareness and understanding of AI-generated content among users to equip them with critical evaluation skills.
Conclusion
Text generation has come a long way, evolving from rudimentary algorithms to sophisticated models capable of generating human-like text. This observational research highlights its multifaceted applications, the current technological landscape, user experiences, and ethical implications. The future of text generation appears promising, yet it requires careful navigation of the associated challenges and ethical considerations.
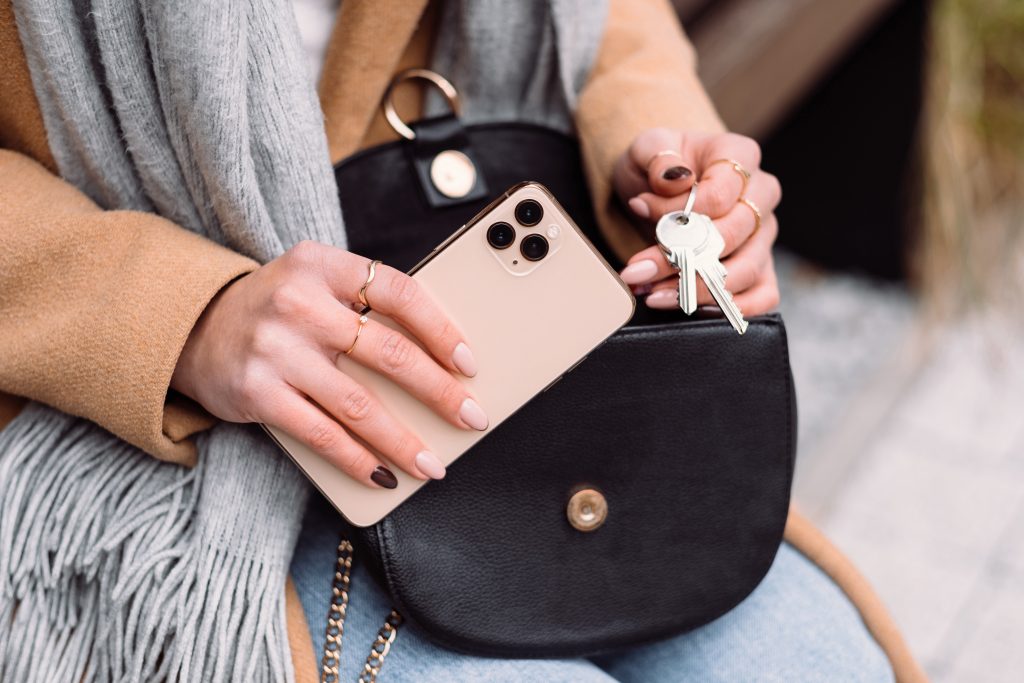